[ad_1]
Constructing accountable AI methods begins with recognizing that know-how options implicitly prioritize effectivity.
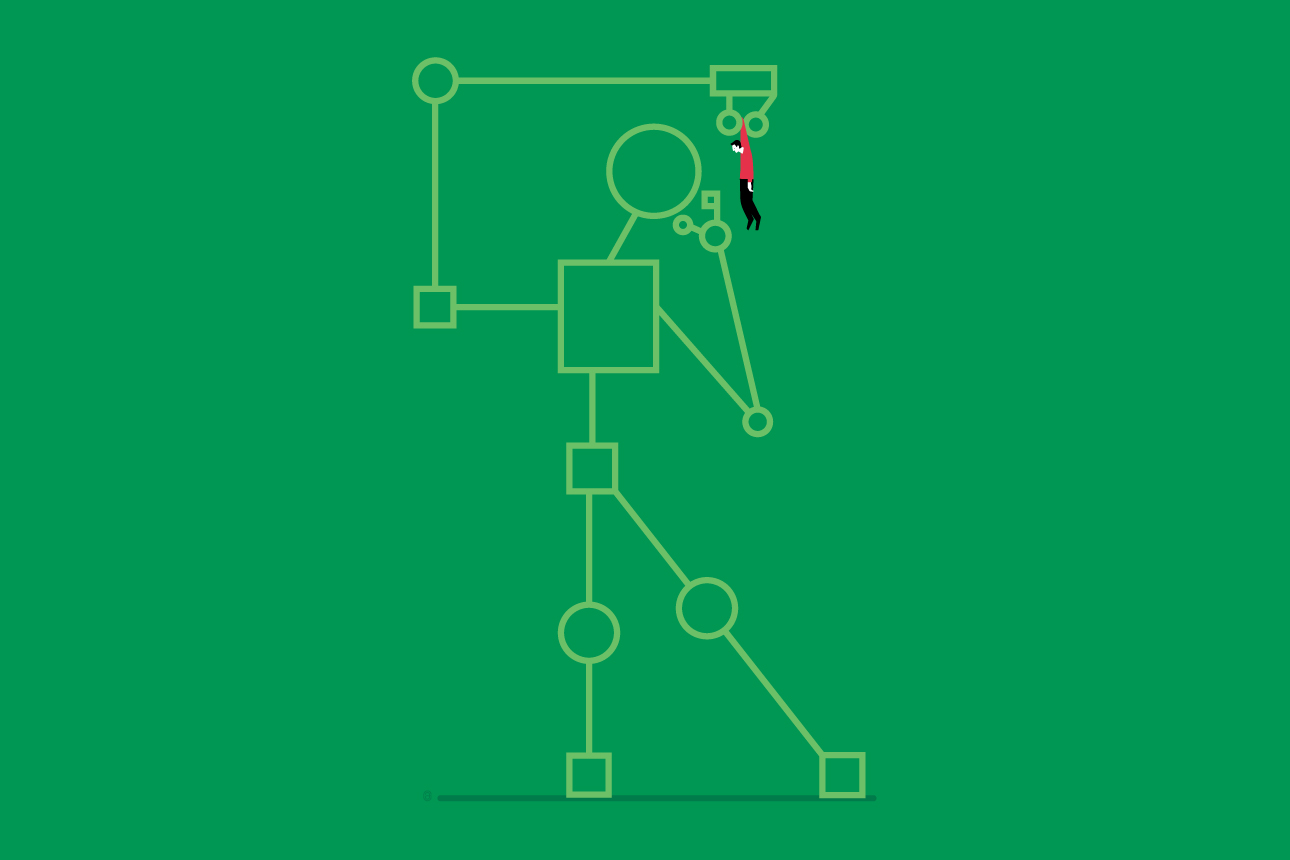
Aad Goudappel/theispot.com
Curiosity within the potentialities afforded by algorithms and massive knowledge continues to blossom as early adopters achieve advantages from AI methods that automate choices as assorted as making buyer suggestions, screening job candidates, detecting fraud, and optimizing logistical routes.1 However when AI purposes fail, they will accomplish that fairly spectacularly.2
Take into account the current instance of Australia’s “robodebt” scandal.3 In 2015, the Australian authorities established its Earnings Compliance Program, with the aim of clawing again unemployment and incapacity advantages that had been made inappropriately to recipients. It got down to establish overpayments by analyzing discrepancies between the annual revenue that people reported and the revenue assessed by the Australian Tax Workplace. Beforehand, the division had used a data-matching method to establish discrepancies, which authorities workers subsequently investigated to find out whether or not the people had actually acquired advantages to which they weren’t entitled. Aiming to scale this course of to extend reimbursements and lower prices, the federal government developed a brand new, automated system that presumed that each discrepancy mirrored an overpayment. A notification letter demanding reimbursement was issued in each case, and the burden of proof was on any people who wished to enchantment. If somebody didn’t reply to the letter, their case was routinely forwarded to an exterior debt collector. By 2019, this system was estimated to have recognized over 734,000 overpayments value a complete of two billion Australian {dollars} ($1.3 billion U.S.).4
Get Updates on Main With AI and Information
Get month-to-month insights on how synthetic intelligence impacts your group and what it means in your firm and clients.
Please enter a sound e mail handle
Thanks for signing up
The brand new system was designed to optimize effectivity, however with out taking note of the particulars of particular person instances. The thought was that by eliminating human judgment, which is formed by biases and private values, the automated program would make higher, fairer, and extra rational choices at a lot decrease value. Sadly, selections made by system designers each in how the algorithm was designed and the way the method labored resulted within the authorities demanding repayments from a whole bunch of hundreds of people that had been entitled to the advantages that they had acquired. Some have been compelled to show that that they had not illegitimately claimed advantages as way back as seven years earlier. The implications for a lot of people have been dire.
Subsequent parliamentary critiques pointed to “a basic lack of procedural equity” and known as this system “extremely disempowering to these individuals who had been affected, inflicting vital emotional trauma, stress, and disgrace.
References
1. T.H. Davenport and R. Bean, “Becoming an ‘AI Powerhouse’ Means Going All In,” MIT Sloan Administration Assessment, June 15, 2022, https://sloanreview.mit.edu.
2. C. O’Neil, “Weapons of Math Destruction: How Large Information Will increase Inequality and Threatens Democracy” (New York: Crown Publishers, 2016).
3. “Accountability and Justice: Why We Need a Royal Commission Into Robodebt,” PDF file (Canberra, Australia: Senate Group Affairs Reference Committee, Might 2022), https://parlinfo.aph.gov.au.
4. “Centrelink’s Compliance Program: Second Interim Report,” PDF file (Canberra, Australia: Senate Group Affairs Reference Committee, September 2020), chap. 1, https://parlinfo.aph.gov.au.
5. “Centrelink’s Compliance Program,” chap. 2.
6. Ibid.
7. D. Lindebaum, C. Moser, M. Ashraf, et al., “Reading ‘The Technological Society’ to Understand the Mechanization of Values and Its Ontological Consequences,” Academy of Administration Assessment, July 2022, https://journals.aom.org.
8. O’Neil, “Weapons of Math Destruction.”
9. M. Rokeach, “The Function of Values in Public Opinion Analysis,” Public Opinion Quarterly 32, no. 4 (winter 1968-1969): 550.
10. O’Neil, “Weapons of Math Destruction.”
[ad_2]
Source link