[ad_1]
Overestimating the capabilities of AI fashions like ChatGPT can result in unreliable functions.
Matters
Frontiers
An MIT SMR initiative exploring how expertise is reshaping the follow of administration.
More in this series
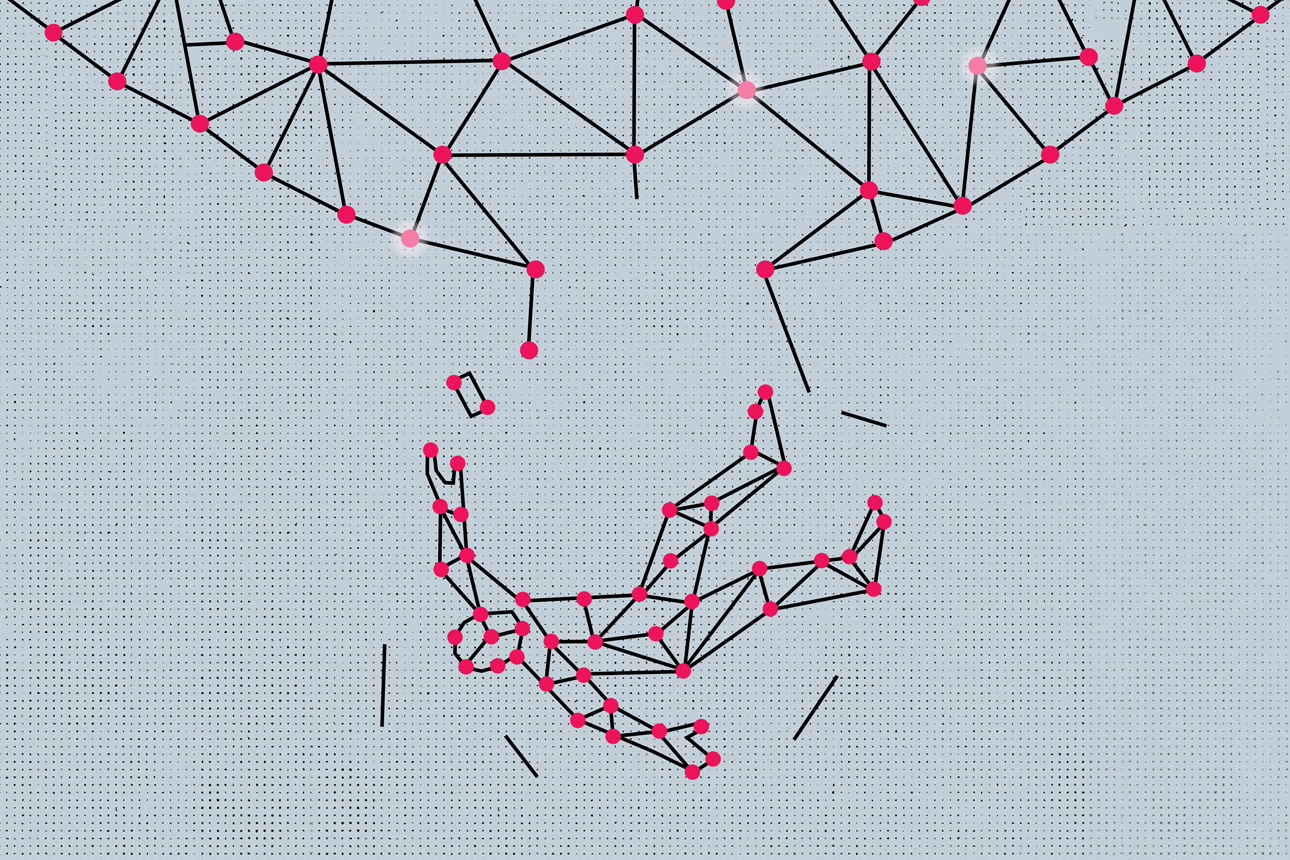
Dan Web page/theispot.com
Massive language fashions (LLMs) appear set to rework companies. Their means to generate detailed, inventive responses to queries in plain language and code has sparked a wave of pleasure that led ChatGPT to achieve 100 million customers quicker than every other expertise after it first launched. Subsequently, traders poured over $40 billion into artificial intelligence startups within the first half of 2023 — greater than 20% of all world enterprise capital investments — and firms from seed-stage startups to tech giants are creating new functions of the expertise.
However whereas LLMs are extremely highly effective, their means to generate humanlike textual content can invite us to falsely credit score them with different human capabilities, resulting in misapplications of the expertise. With a deeper understanding of how LLMs work and their basic limitations, managers could make extra knowledgeable selections about how LLMs are used of their organizations, addressing their shortcomings with a mixture of complementary applied sciences and human governance.
Get Updates on Main With AI and Information
Get month-to-month insights on how synthetic intelligence impacts your group and what it means in your firm and clients.
Please enter a legitimate e mail handle
Thanks for signing up
The Mechanics of LLMs
An LLM is essentially a machine studying mannequin designed to foretell the following component in a sequence of phrases. Earlier, extra rudimentary language fashions operated sequentially, drawing from a chance distribution of phrases inside their coaching knowledge to foretell the following phrase in a sequence. (Consider your smartphone keyboard suggesting the following phrase in a textual content message.) Nonetheless, these fashions lack the power to think about the bigger context wherein a phrase seems and its a number of meanings and associations.
The arrival of the newest neural community structure — transformers — marked a big evolution towards fashionable LLMs. Transformers permit neural networks to course of giant chunks of textual content concurrently as a way to set up stronger relationships between phrases and the context wherein they seem. Coaching these transformers on more and more huge volumes of textual content has led to a leap in sophistication that permits LLMs to generate humanlike responses to prompts.
This means of LLMs hinges on a number of essential elements, together with the mannequin’s measurement, denoted by the variety of the trainable weights (often known as parameters), the standard and quantity of the coaching knowledge (outlined by variety of tokens, referring to phrase or subword items), and the utmost measurement of enter the mannequin can settle for as a immediate (often known as its context window measurement).
[ad_2]
Source link