[ad_1]
Machine studying options have already automated a big a part of how the world used to function and are taking care of their very own inefficiencies now. So sure, the info science world will not be immune from the vantage of automation and is witnessing core machine studying engineering processes getting automated to allow smoother and sooner improvement.
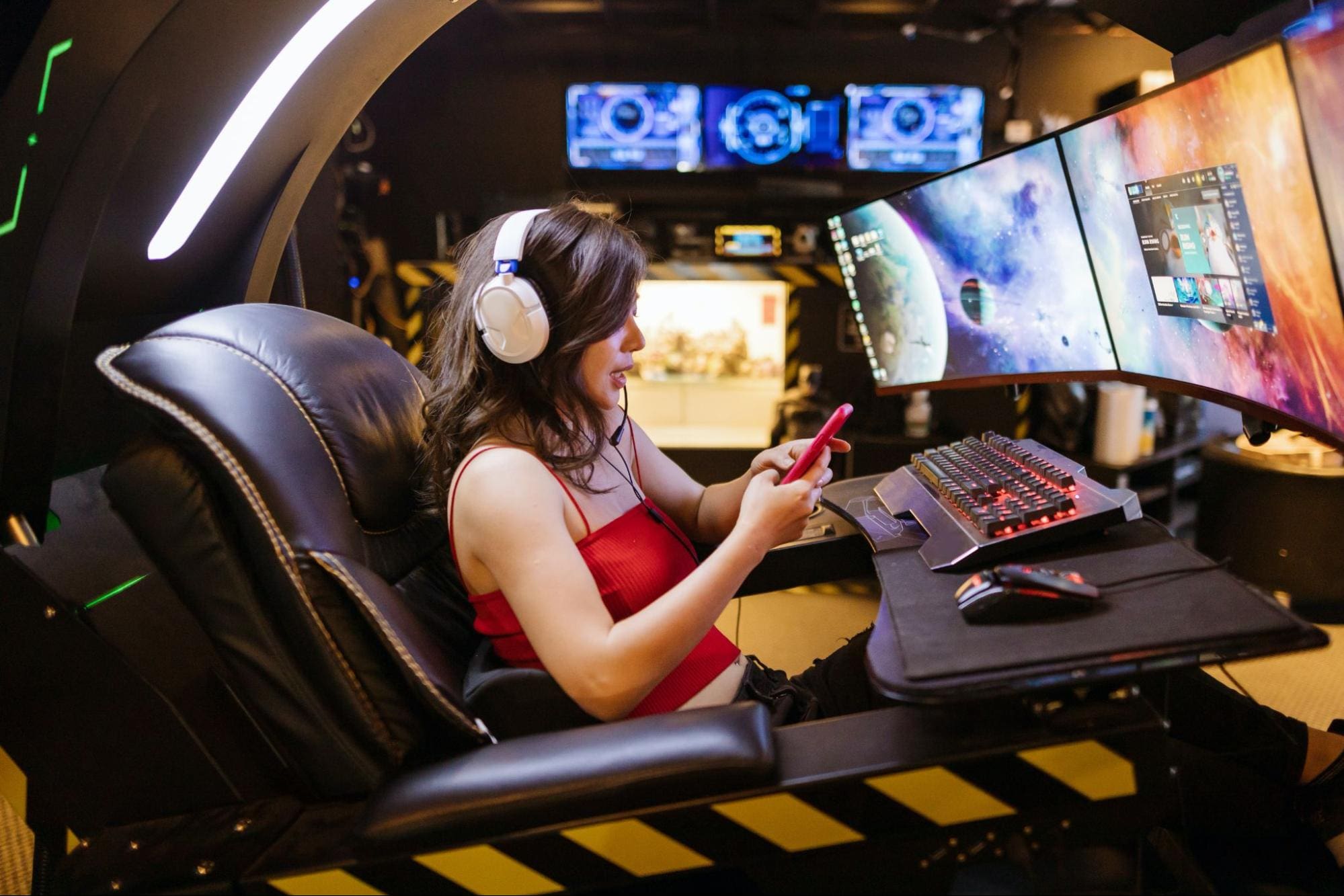
Picture by RODNAE Productions
Consider the instances when a number of steps – from knowledge integration to mannequin coaching, choice, and deployment – have been accomplished manually. Every step may be very rigorous and requires intensive effort from knowledge scientists. Inarguably, automation turns into extremely valued in serving to knowledge scientists via end-to-end modeling and deployment processes.
Automated Machine Studying (AutoML) considerably boosts the developer’s productiveness, permitting them to give attention to the important thing modeling areas that require their time and a spotlight.
Earlier than we assess the professionals and cons of AutoML, allow us to first perceive how the info science world used to operate previous to the automation of machine studying processes to know its worth proposition higher.
AutoML is commonly seen within the mild of replicating knowledge scientists’ work however is reasonably an enabler for constructing higher fashions sooner. There’s a gamut of issues which are nonetheless accomplished manually by knowledge scientists and pose challenges to machine studying implementation. Ryohei Fujimaki, the CEO of dotData, explains as follows.
It’s vital for organizations to not view automation as a “alternative” for knowledge scientists however as an alternative as a software of the commerce. We have discovered that many enterprises now divide the function engineering course of out of the info science group and into devoted teams that concentrate on function discovery. Whatever the setup, offering automation instruments and platforms to make the info scientist’s job simpler must be the main target.
– Ryohei Fujimaki, the CEO of dotData
One of the crucial vital but very essential and time-consuming steps of a machine studying pipeline is knowledge evaluation and testifying to good high quality knowledge. Any failure or deviation to element at this step can price you closely and thus requires a talented knowledge analyst to set the foundations proper.
Apart from knowledge evaluation, knowledge cleansing and have engineering give a major raise to the mannequin to study the phenomenon a lot sooner. However the caveat is that these expertise are constructed over time. So, as an alternative of ready to construct the fitting group and expertise to sift patterns via the humongous datasets and generate beneficial insights, the automation of machine studying workflows removes the limitations to constructing fashions.
Put merely, it helps enterprises rapidly scale their machine-learning initiatives by enabling non-technical consultants to leverage the ability of such refined algorithms. Not solely does automation assist enhance the mannequin accuracy, however it additionally brings the business’s finest practices so nobody has to reinvent the wheel on already solved areas involving repetition.
Sparing knowledge scientists the time spent on infinite trivial duties that may be simply automated, empowers them with the mind energy to convey innovation to life.
Referring to Microsoft’s view on AutoML, it’s the strategy of automating the time-consuming, iterative duties to construct ML fashions with giant scale, effectivity, and productiveness all whereas sustaining mannequin high quality.
It requires a mindset shift to reinforce the processes and construct methods via automating handbook duties similar to function engineering, function discovery, mannequin choice, and extra.
The information science course of continues to be a largely handbook endeavor. Utilized correctly, automation can present knowledge scientists a substantial amount of help with out having to concern ‘job losses.’ When AutoML first grew to become in style, the dialogue within the DS neighborhood was largely in regards to the professionals and cons of automating your complete life cycle of the info science course of. At dotData, we have discovered that such an “all or nothing” strategy underestimates the complexity of the info science course of – particularly in giant organizations. Because of this, we consider that corporations ought to focus as an alternative on offering automation, which makes the lifetime of the info scientist less complicated and their job simpler. One such space is function engineering. Knowledge scientists spend an inordinate period of time working with knowledge engineers and subject material consultants to find, develop and optimize the absolute best options for his or her fashions. By automating a big a part of the function discovery course of, knowledge scientists can give attention to the duty they’re really designed to carry out: constructing the absolute best ML fashions.
– Ryohei Fujimaki, the CEO of dotData
Apart from boosting productiveness and effectivity, it additionally alleviates the chance of human errors and biases which provides to mannequin reliability. However, as consultants say, extra of all the things is unhealthy. So, automation may be finest utilized when assisted with some extent of human oversight to think about real-time info and area experience.
Now that we perceive the advantages of automation, allow us to zoom in on the precise steps and processes which are most wieldy of effort and time. Automation within the areas listed beneath has the potential to make a noteworthy enhance in effectivity in addition to accuracy:
- Knowledge Preparation: Knowledge coming from disparate sources makes it a difficult activity for the info scientists to arrange it in the fitting format to enter to the mannequin coaching stage. It entails a mess of steps similar to knowledge assortment, cleansing, and preprocessing to call a couple of.
- Characteristic Choice and Characteristic Engineering: Deciding on and presenting the fitting options to mannequin is foundational to studying the fitting phenomenon. Not solely does automation helps to find the fitting options, however it is usually used to engineer new options to speed up the training course of.
- Mannequin Choice: It’s the strategy of discovering the best-performing mannequin among the many set of candidate fashions and governs the accuracy in addition to the robustness of the mannequin improvement pipeline. AutoML may be very helpful in iterating and figuring out the fitting mannequin for the given activity.
- Hyperparameter Optimization: Deciding on the fitting mannequin will not be ample, you additionally want to search out the fitting hyperparameters for a given machine studying algorithm similar to studying charge, variety of layers, and variety of epochs. Such mannequin settings require a machine studying engineer to tune these parameters that optimally clear up the machine studying drawback. An automatic hyperparameter optimization is an indispensable software that finds the very best structure to your mannequin by assessing varied combos.
- Mannequin Monitoring: No machine studying mannequin is ready to proceed giving correct predictions with out the necessity for periodic retraining. Automated instruments monitor and set off the mannequin pipeline to take corrective actions if the deployed mannequin deviates from the anticipated efficiency.

Picture from Canva
Automation, usually, is dreaded as “expertise taking away jobs”, nonetheless, it primarily helps in streamlining repetitive and mundane duties. Automation in knowledge science is an enormous enabler for knowledge scientists by reducing down on handbook efforts thereby permitting for improved and environment friendly modeling processes. One should complement AutoML with truthful participation of human experience and oversight to get the complete advantages of automating the difficult components of knowledge science workflows.
Vidhi Chugh is an AI strategist and a digital transformation chief working on the intersection of product, sciences, and engineering to construct scalable machine studying methods. She is an award-winning innovation chief, an creator, and a world speaker. She is on a mission to democratize machine studying and break the jargon for everybody to be part of this transformation.
[ad_2]
Source link