[ad_1]
Most AI/machine studying initiatives report solely on technical metrics that don’t inform leaders how a lot enterprise worth could possibly be delivered. To forestall challenge failures, press for enterprise metrics as an alternative.
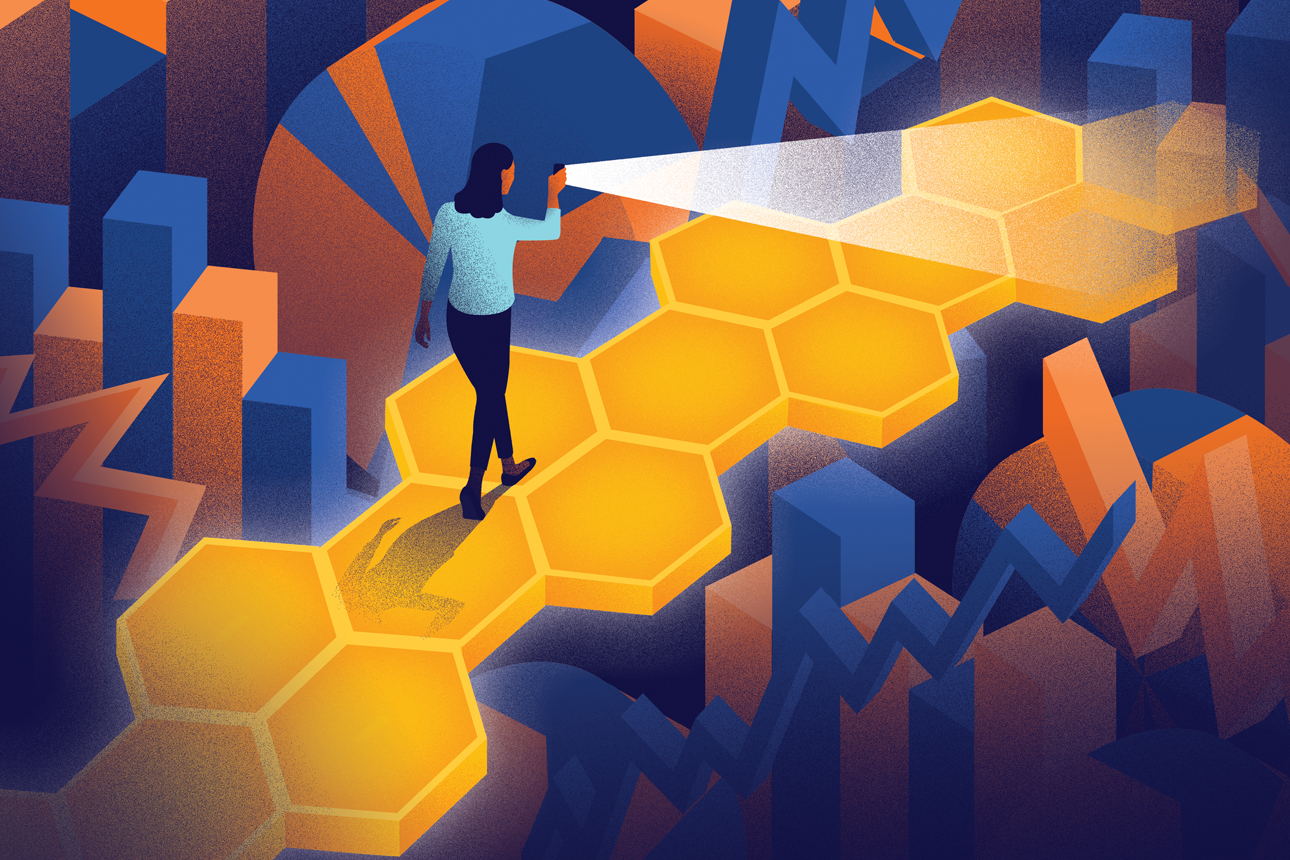
Neil Webb/theispot.com
“AI” can imply many issues, however for organizations utilizing synthetic intelligence to enhance present, large-scale operations, the relevant know-how is machine studying (ML), which is a central foundation for — and what many individuals imply by — AI. ML has the potential to enhance all types of enterprise processes: It generates predictive fashions that enhance focused advertising, fraud mitigation, monetary threat administration, logistics, and way more. To distinguish from generative AI, initiatives like these are additionally generally referred to as predictive AI or predictive analytics. You would possibly anticipate that the efficiency of those predictive ML fashions — how good they’re, and the way a lot worth they ship — can be entrance and heart. In any case, producing enterprise worth is the entire level.
However you’ll be mistaken. In terms of evaluating a mannequin, most ML initiatives report on the mistaken metrics — and this typically kills the challenge totally.
Get Updates on Main With AI and Knowledge
Get month-to-month insights on how synthetic intelligence impacts your group and what it means to your firm and prospects.
Please enter a legitimate e mail tackle
Thanks for signing up
On this article, tailored from The AI Playbook: Mastering the Rare Art of Machine Learning Deployment, I’ll clarify the distinction between technical and enterprise metrics for benchmarking ML. I’ll additionally present how you can report on efficiency in enterprise phrases, utilizing bank card fraud detection for instance.
Why Enterprise Metrics Should Come First
When evaluating ML fashions, knowledge scientists focus nearly totally on technical metrics like precision, recall, and raise, a form of predictive multiplier (in different phrases, what number of occasions higher than guessing does the mannequin predict?). However these metrics are critically inadequate. They inform us the relative efficiency of a predictive mannequin — compared to a baseline comparable to random guessing — however present no direct studying on absolutely the enterprise worth of a mannequin. Even the commonest, go-to metric, accuracy, falls into this class. (Additionally, it’s usually impertinent and often misleading.)
As an alternative, the main focus must be on enterprise metrics — comparable to income, revenue, financial savings, and variety of prospects acquired. These simple, salient metrics gauge the basic notions of success. They relate on to enterprise goals and reveal the true worth of the imperfect predictions ML delivers. They’re core to building a much-needed bridge between business and data science teams.
Sadly, knowledge scientists routinely omit enterprise metrics from experiences and discussions, regardless of their significance. As an alternative, technical metrics dominate the ML follow — each by way of technical execution and in reporting outcomes to stakeholders.
[ad_2]
Source link